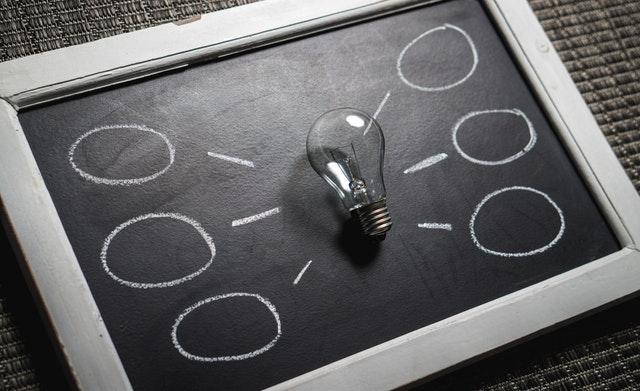
Avoid these common machine learning mistakes
Big players like Facebook, YouTube, Amazon, Google and Netflix are experts in using this technology and avoiding machine learning mistakes. Using machine learning has become attractive for every industry. I have already shared one of my previous articles on how machine learning has reshaped the business environment.
How can the beginners in this area avoid making machine learning mistakes when implementing these projects in their day to day activities?
Strategy first; Discuss and plan how the outcomes add real value to the business. In some cases, when a company already has strong analytics platforms, there is no need for a machine learning implementation. Not all use cases require this kind of technology, there are cases when this technology has been implemented with no positive effect on the business.
It is better to plan a machine learning project with data scientists who are experts in computer science, mathematics and domain expertise. An alternative could also be having a user-friendly data visualization tool which can be explored by others within the company.
The quality of data is a significant when dealing with machine learning. It’s essential to have good and clean data to obtain relevant and accurate results. So, when you start your strategy, this is one of the main aspects to think about.
Infrastructure is another mistake that can be easily avoided if we consider the fact that our database management systems can be unprepared for such a significant volume of data machine learning needs. We need to establish flexible storage that will be able mature as the technologies progress. This translates into powerful computation and elasticity that is critical when when setting up your machine learning infrastructure.
If you already use machine learning remember to analyze the data upfront and then design the algorithms, have your internal stakeholders on the project avoid these most common mistakes below;
- Use low function carefully and don’t take it for granted
- Don’t use plain linear models for non-linear interaction
- Remember outliers are very relevant and exciting – sometimes they need special attention, and sometimes they should be completely ignored
- During any machine learning project it is very important to determine success metrics
- Even if you set the right objective, be careful to plan the right way to reach it
Machine learning methods requires complex projects that need special attention for avoiding the typical machine learning mistakes that can influence the effectiveness and outcome. Learn from the mistakes that have already been made by others.
Photo source: Pexels.com